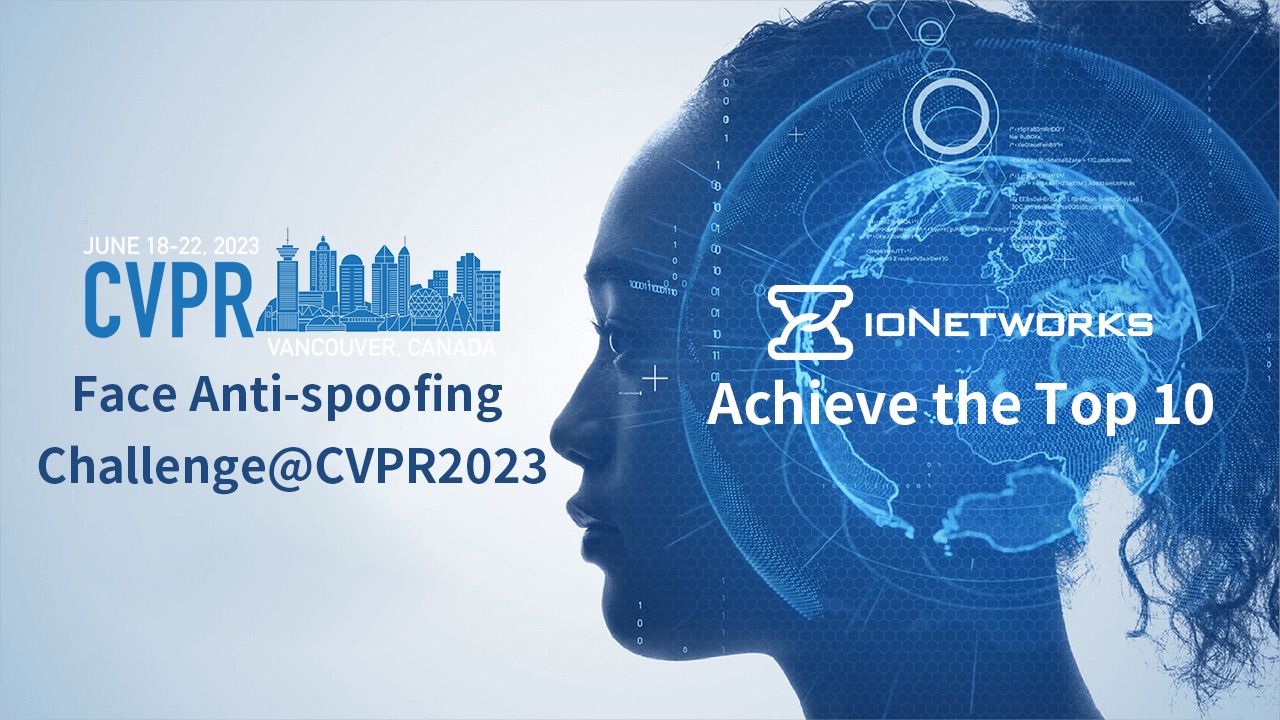
ioNetworks’ EZ Face Recognition AI facial recognition algorithm performed well in the Face Anti-spoofing Challenge at the Computer Vision and Pattern Recognition Conference (CVPR) hosted by the IEEE Computer Society in March 2023. Using traditional RGB 2D camera, the model achieved an AUC performance indicator of over 95% in the anti-spoofing test, making ioNetworks the only Taiwanese developer to enter the top ten. In the future, this technology will be implemented in edge computing devices such as eKYC, autonomous vehicles, smart security systems, smart home, computer, gaming, conference peripherals and access control.
The act of forging a human face, including using photos, videos, masks, and other means of attack, for identity deception and system invasion, not only causes losses but also limits the development of facial recognition applications. The "Face Anti-spoofing Challenge" aims to promote the evolution of facial anti-spoofing technology. Participants are required to develop a solution that can detect and distinguish between real and forged faces. In the past, commonly used 2D facial anti-spoofing detection technology required users to make movements such as blinking or turning their heads in order to detect spoofing. However, this competition requires the direct use of 2D image information for recognition.
The solution submitted by ioNetworks is based on deep learning and artificial intelligence technology, using convolutional neural networks (CNN) to distinguish between real and fake faces. In order to improve the efficiency of image information utilization, this solution adopts a model structure with tight connections from shallow to deep layers, and at the same time, improves the accuracy of the algorithm through data augmentation.
In addition, ioNetworks does not view the face anti-spoofing task as a traditional binary classification problem. By incorporating supervised training methods at the regional level, the model can further use local facial details to judge authenticity. Finally, this solution introduces loss functions developed in the field of face recognition research, which can effectively reduce the impact of data noise on the algorithm and obtain more stable face anti-spoofing prediction results.
In today's society, problems such as identity fraud and system hacking have become increasingly serious. The use of facial recognition technology can help improve security. However, the development of fake face technology has made this field more challenging. Through such competitions, participants can explore and develop more advanced facial anti-spoofing technologies together, contributing to the improvement of network security and the prevention of identity fraud and other issues.
About CVPR
CVPR is abbreviated as "Computer Vision and Pattern Recognition Conference", which is one of the top international conferences in the field of computer vision and pattern recognition. Every year, scholars, researchers, and industry experts around the world attend the conference. The main goal of CVPR is to promote the development and advancement of computer vision and pattern recognition, and to provide a platform for communication and discussion.
CVPR is organized by IEEE Computer Society and was first held in 1983. It has now become one of the top conferences in the field. At CVPR, researchers can present their research findings through keynote speeches, technical reports, and poster presentations, and also have the opportunity to communicate and collaborate with other scholars.
The main research fields of CVPR include computer vision, pattern recognition, image processing, deep learning, 3D reconstruction, and more. The development in these areas is important for the advancement of machine vision, autonomous driving, artificial intelligence, robotics, and other related fields.
Related Products
Turing Series-EZ Match Face
EZ Match Face is a facial recognition software developed based on AI artificial intelligence. It has the best user experience and the fastest deployment, and can be integrated into the EZ Pro image management platform or executed separately.